A Digital Twin Approach for Battery Management Systems for LiFePO4 Batteries
A Digital Twin Approach for Battery Management Systems for LiFePO4 Batteries Roger Painter 1,5, Ranganathan Parthasarathy 1, Lin Li 1, Irucka Embry 2, Lonnie Sharpe 3 and S. Keith Hargrove 4 1 Department of Civil Engineering, Tennessee State University, Nashville, TN 37209, USA; rpainter@tnstate.edu; rparthas@tnstate.edu; lli1@tnstate.edu 2 EcoC²S, Nashville, TN 37206, USA; iembry@my.tnstate.edu 3 Department of Mechanical Engineering, Tennessee State University, Nashville, TN 37209, USA; sharpe@coe.tsuniv.edu 4 Tuskegee University, Tuskegee, AL 36088, USA; skhargrove@gmail.com 5 Correspondence: rpainter@tnstate.edu
The emerging digital twin vision for computer simulation encompasses the entire lifecycle of real-world applications and products. The digital twin concept enables the integration of complex multiphysics and multidimensional models and simpler reduced order models (ROM). The multiphysics models store current expert engineering knowledge that is accessible and reusable by non-experts at all stages of the products lifecycle via locally implemented or on-board ROM. Furthermore, the ROM can provide real time operational and control capabilities that are subject to verification and updating by the off board multiphysics model. Our approach to developing a digital twin simulation of lithium-ion batteries for electric vehicles utilized a dataset generated from the COMSOL® Multiphysics simulation of the Cahn–Hilliard equation for a single particle model (SPM) of a lithium iron phosphate (LiFePO4) cathode. The SPM cannot simply be scaled up to bulk scale processes, so we took a machine learning (ML) approach to derive latent variables from the data. We relate the SPM simulation statistically to the battery cell voltage and temperature responses. Specifically, the plateauing effect of the battery’s voltage response at higher ambient temperatures and the apparent diffusion-controlled behavior at lower temperatures are related by statistical inference to the SPM. The simulation dataset was first subjected to singular value decomposition (SVD) and principal component analysis (PCA) to identify latent variables and for model order reduction. The ROM was then developed by utilizing the reduced order simulation dataset and experimental data for an A123 Systems 26650 2.3 Ah cylindrical battery to train a self-normalizing neural network (SNN). Finally, the ROM was verified as an on-board battery management system (BMS) for ambient temperatures ranging from 253 to 298 K and discharge rates ranging from 1 C to 20.5 C.
Keywords: lithium-ion batteries; LIB; LiFePO4; electric vehicles; Cahn–Hilliard; principal component analysis, neural networks
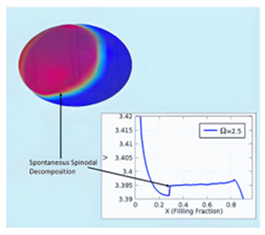