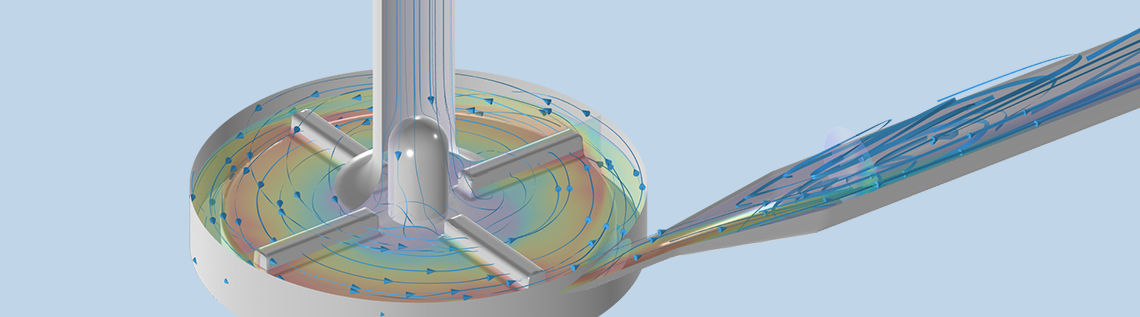
Computational fluid dynamics (CFD) modeling is an effective tool for modeling fluid flow in cardiovascular applications and devices. It has been used extensively to simulate hemodynamics within blood-contacting medical devices. Using CFD modeling for virtual testing in place of physical testing methods allows device developers to accelerate the development cycle, reduce costs, and validate device safety and effectiveness without exposing patients to unproven medical device prototypes.
Because of these benefits, the U.S. Food and Drug Administration (FDA) routinely advises the industry on using modeling and simulation to predict clinical outcomes, inform clinical trial designs, and support evidence of effectiveness (Ref. 1). In an effort to improve and standardize the use of CFD modeling for the regulatory submissions of cardiovascular devices, the FDA developed two benchmark models of the fluid flow in a nozzle and a centrifugal blood pump. To validate CFD models, in vitro experiments were performed in multiple labs to obtain experimental velocities, pressures, and hemolysis data. CFD modeling of the nozzle benchmark was previously done by Veryst Engineering, a COMSOL certified consultancy.
In this blog post, we present the centrifugal blood pump model using the COMSOL® software.
The Model
We obtained the pump geometry from the National Cancer Institute’s website and prepared it for CFD modeling in the COMSOL® software according to the specifications provided by the FDA. We used the CFD Module and Mixer Module in the COMSOL® software to run a frozen rotor study, which required the fluid domain to be divided into a stationary and a rotating subdomain. Geometry cleanup and modification were kept minimal.
Model definition for the centrifugal blood pump.
A Newtonian fluid was used to describe blood according to the FDA guidelines. The k-epsilon turbulence model was used to obtain a good initial fluid flow solution for the shear stress transport (SST) model, which was subsequently used to obtain flow solutions with higher fidelity. Flow rates of 2.5–7 l/min at a pump speed of 3500 rpm were simulated.
A frozen rotor study was used to compute the (pseudo) steady-state solution.
Validation
The FDA published its benchmark centrifugal blood pump study in 2017, reporting results from computational studies as well as the data from its in vitro experiments (Ref. 2). We extracted the experimental data from the graphs in the publication and compared it to the results of our CFD model in order to validate the CFD results.
Pressure head across the pump was computed at a pump speed of 3500 rpm for several flow rates. The computed results match the physical measures well, as you can see in the following graph.
A graph showing the results of the pressure head at several different flow rates for the centrifugal pump functioning at 3500 rpm.
Two-dimensional velocity magnitude at a pump condition of 6 l/min and 3500 rpm was computed at the upper blade plane. The computed radial velocity magnitudes match the measurements qualitatively and are consistent with what was reported by other CFD studies (Fig 6A,C of Ref. 2).
A graph showing the velocity magnitude inside the blood pump model based on the x and y velocity components along the radial cut line. The graph also shows a comparison of the model’s results with the experimental results from Malinauskas et. al. (Ref. 2).
Similarly, the velocity profile was computed at x = 0.035 m in the diffuser region at a pump condition of 6 l/min and 3500 rpm. The computed velocity magnitudes match the measurements qualitatively and are consistent with what was reported by other CFD studies (Fig 6B,D of Ref. 2).
A graph showing the velocity magnitude inside the blood pump based on the x and y velocity components along the diffuser cut line. The graph also shows a comparison of the model’s results with the experimental results from Malinauskas et. al. (Ref. 2).
The model file serves as a demonstration of how COMSOL Multiphysics makes postprocessing easy. Users can set up probes and non-local coupling operators (e.g., average, surface integration, etc.) to calculate derived values from computed CFD results. It is also very convenient to extract data subsets from the CFD dataset with various types of cut operations.
Finally, we plotted the interpolated contours of three-dimensional velocity magnitude for the upper-blade passage plane at a pump condition of 6 l/min and 3500 rpm. The results match qualitatively with other CFD studies reported in Ref. 2.
Visualization of rotation and velocity slice plots with Transformation 3D dataset.
Verification and Validation (V&V)
Acceptance of modeling and simulation in medical device evaluation requires adequate verification, validation, and uncertainty quantification (VVUQ). The ASME V&V 40 standard (Ref. 3) was published in 2018 and provides a framework for assessing the credibility of computational models of medical devices, with the devices’ risk factors taken into account. The key concept for model risk evaluation is context of use (COU). In the framework recommended by V&V 40, the “model risk is a combination of the influence of the computational model relative to other contributing evidence for making a decision, and the consequence for the patient or end users if a decision is incorrect.” In other words, the credibility should be commensurate with the degree to which the computational model is relied upon as evidence. For a left ventricular assist device (LVAD) such as the blood pump, an incorrect credibility decision would be detrimental to the patient. Therefore, the decision consequence associated is ranked “high” (Ref. 3).
An important aspect of V&V is code verification. The COMSOL Verification and Validation Models web page provides more than 140 verification and validation examples available for download. In the area of fluid flow, COMSOL models have been verified with analytical solutions as well as with scientific publications that solve the same equations, and validated using widely accepted experimental data.
We have also released the Uncertainty Quantification Module in COMSOL Multiphysics version 6.0. The goal is to provide COMSOL users with a UQ tool that easily integrates with COMSOL Multiphysics models. In biomedical applications, real model parameters are usually not accurately known. Variability is also inherent in living organisms. We hope that it is helpful for medical device modelers to investigate how the quantities of interest depend on variations in the inputs of a model.
Conclusion
Computational fluid dynamics (CFD) can be effectively used to characterize fluid flow in medical devices. In this blog post, we presented a CFD solution for the FDA blood pump benchmark model using the CFD Module and Mixer Module. The two modules offer an effective CFD solution for fluid flow in rotating machinery, common in centrifugal blood pumps. The results are consistent with the FDA’s reported studies, both experimental and computational.
Try It Yourself
The FDA blood pump model demonstrates how COMSOL Multiphysics makes postprocessing easy. Try it yourself by clicking the button below, which will take you to the Application Gallery entry.
Interested in Other Biomedical Use Cases for Simulation?
Check out these resources to see how the COMSOL Multiphysics has been used to model various biomedical applications:
- The COMSOL News Special Edition Biomedical
- The proceedings of a 2021 COMSOL Day on biomedical devices
References
- T. Morrison, “How Simulation Can Transform Regulatory Pathways”, U.S. Food & Drug Administration, 9 Aug. 2018; https://www.fda.gov/science-research/about-science-research-fda/how-simulation-can-transform-regulatory-pathways
- R. A. Malinauskas, P. Hariharan, S. W. Day, L. H. Herbertson, M. Buesen, U. Steinseifer and B. A. Craven, “FDA benchmark medical device flow models for CFD validation”, Asaio Journal, 63(2), 150–160, 2017; https://pubmed.ncbi.nlm.nih.gov/28114192/
- ASME, “Assessing Credibility of Computational Modeling through Verification and Validation: Application to Medical Devices”, 2018; https://www.asme.org/codes-standards/find-codes-standards/v-v-40-assessing-credibility-computational-modeling-verification-validation-application-medical-devices
Comments (0)